Machine learning and materials simulation are aiding in designing more reliable materials, especially in applications where high temperatures and mechanical stress are the norms. A research team led by Lehigh University has developed an innovative approach for predicting abnormal grain growth in polycrystalline materials well before it becomes apparent. The research, published in Nature Computational Materials can be found here:
Zhou, H., Zalatan, B., Stanescu, J., Harmer, M. P., Rickman, J. M., He, L., Marvel, C. J., & Chen, B. Y. (2025). Learning to predict rare events: the case of abnormal grain growth. Npj Computational Materials, 11(1), 82. https://doi.org/10.1038/s41524-025-01530-8
The research team used a novel simulation framework that integrates two machine learning techniques. They combined a long short-term memory (LSTM) network with a graph-based convolutional network (GCRN) in what they refer to as the Predicting Abnormality with GCRN and LSTM (PAGL) framework. This integrated method is able to analyse the evolving microstructure of materials and detect subtle changes in the early stages of grain development. The early detection system was not expected. Dr. Brian Y. Chen, an associate professor at Lehigh University’s P.C. Rossin College of Engineering and Applied Science stated:
“We thought that the data might be too noisy, maybe the properties we were looking at wouldn’t reveal very much about distant future abnormalities, or maybe the abnormality would only reveal itself just as it was about to happen, when it might be obvious even to the human eye. But we were surprised that we were actually able to make predictions so far in advance.”
Critical to that early detection was using their models to examine the grain’s characteristics over time before the abnormality occurred.
“A better way to think about grains becoming abnormal is to think about how they evolve in the time before they change, so at 10 million time steps before abnormality, for example, they have certain properties that might differ from those they had at 40 million time steps.”
Chen also explains that the team’s method can predict abnormal grain growth during only the first 20% of the simulated material lifetime in 86% of the cases. This early detection is critical, as abnormal grain growth; where certain crystalline grains in a material increase disproportionately, can lead to brittleness, reducing a component’s performance under stress. Chen said:
“Using simulations, we were not only able to predict abnormal grain growth, but we were able to predict it far in advance of when that growth happens,”
Predicting which alloy compositions might develop abnormal grain growth has been compared to searching for a needle in a haystack, given the myriad of possible material combinations and processing conditions. Conventional methods required lengthy simulations and extensive experimental testing, but the new machine learning model streamlines the process by quickly eliminating unsuitable candidate materials. The framework could help cut down on both time and resources during the material selection phase.
“Our results are important because if you want to look at that big haystack of different materials, you don’t want to have to simulate each one for too long before you know whether or not abnormal grain growth is going to occur, you want to simulate for as little time as possible, and then move on.”
The team employed a modified 3D Monte Carlo Potts simulation to model microstructural changes over time. These types of simulations take into account the movement of atoms within the material as they are subjected to high temperatures; a common scenario in rocket engines and high. performance combustion systems. By integrating the simulation outputs with the LSTM and GCRN models, the researchers were able to map out the evolution of each grain and its likelihood of deviating from normal growth behavior.
Dealing with the inherent rarity of abnormal grain growth events was incredibly important within the research. In the early stages of simulation, grains destined to grow abnormally do not differ noticeably from their neighbours. However, by focusing on their gradual evolution rather than waiting for an obvious change, the team’s approach has provided an effective early-warning system. Martin Harmer, Lehigh’s Alcoa Foundation Professor of Materials Science and Engineering, Emeritus; director of the Nano/Human Interface Presidential Research Initiative; and co-author of the paper stated:
“This work opens up an exciting new possibility for material scientists to ‘look into the future’ to predict the future evolution of material structures in ways that were never possible before, it will have a major impact in designing reliable materials for defense, aerospace and commercial applications.”
The research was authored by Houliang Zhou, Benjamin Zalatan, Jacob Stanescu, Martin P. Harmer, John M. Rickman, Lei He, Christopher J. Marvel, and Brian Y. Chen, who together developed and validated the PAGL framework for early detection of grain abnormalities.
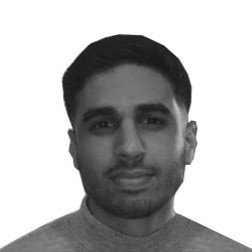
Hassan graduated with a Master’s degree in Chemical Engineering from the University of Chester (UK). He currently works as a design engineering consultant for one of the largest engineering firms in the world along with being an associate member of the Institute of Chemical Engineers (IChemE).