New emission standards such as the European Union’s proposed Euro 7 requires catalysts that can efficiently convert nitrogen oxides (NOₓ) at lower exhaust temperatures. Researchers at Chalmers University of Technology, together with industrial partner Umicore and the Technical University of Darmstadt, have developed a machine learning based force field to model copper-exchanged chabazite (Cu-CHA) catalysts and their interaction with ammonia under realistic conditions. The research was published Nature Communications and can be found here:
Bjerregaard, J.D., Votsmeier, M. & Grönbeck, H. Influence of aluminium distribution on the diffusion mechanisms and pairing of [Cu(NH3)2]+ complexes in Cu-CHA. Nat Commun 16, 603 (2025). https://doi.org/10.1038/s41467-025-55859-1
Selective catalytic reduction (SCR) uses ammonia to transform NOₓ into nitrogen and water in an oxygen-rich exhaust stream. An effective SCR catalyst must promote the formation of an N–N bond between NOₓ and NH₃ while suppressing side reactions; such as oxidation of ammonia to N₂O or additional NOₓ, that would reduce overall efficiency.
Chabazite zeolites, with their network of cages and channels, become active SCR catalysts when copper ions replace protons in the framework. Under low-temperature conditions, each Cu²⁺ site binds two ammonia molecules, forming mobile [Cu(NH₃)₂]⁺ complexes that diffuse through the cages. The key step in SCR is when two of those complexes meet in the same cage, enabling the NOₓ reduction reaction.
The dynamic behaviour of these solvated complexes poses a challenge for atomistic models, which usually assume that intermolecular forces are strictly local and cannot account for long-range Coulomb interactions critical in zeolites. To overcome this, the team trained a machine learning force field on extensive first-principles calculations, explicitly incorporating long-range electrostatics to capture the diffusion of charged complexes. Henrik Grönbeck, Professor at the Department of Physics, Chalmers University of Technology and one of the key authors of the study said:
“Because of the highly dynamic character of the catalyst, computational investigations are important to understand how detailed structure and composition influence performance, In our recent study we have developed a machine learning force field—a computational model that is used to describe the forces between atoms. Our force field includes long-range electrostatic interactions, which makes it possible to study the diffusion of the charged ammonia–copper–ammonia complexes.”
Using this Machine Learning force field, the researchers mapped how variations in aluminium placement within the zeolite framework affect both the mobility of [Cu(NH₃)₂]⁺ and the likelihood of pairing. They found that regions with paired aluminium sites lower the barrier for two complexes to occupy the same cage, a configuration essential for efficient NOₓ conversion. Joachim Bjerregaard, a doctoral student at the Department of Physics and main author stated:
“Using the machine learning force field it has been possible to reveal the atomistic mechanisms for charged ammonia–copper–ammonia diffusion and understand how the composition of the material affects the formation of charged ammonia–copper–ammonia pairs and their stability,”
This research could provide a way of synthesizing real catalysts; by controlling aluminium distribution and copper loading to maximise the formation and stability of reactive [Cu(NH₃)₂]⁺ pairs. Beyond SCR, the approach may also have uses to other zeolite-based processes, including one-step conversion of CO₂ to methanol or longer hydrocarbons. Grönbeck stated:
“The application of accurate machine learning force fields to zeolite systems is an exciting development that helps us to accelerate the understanding of complex systems and suggest new efficient catalytic materials,”
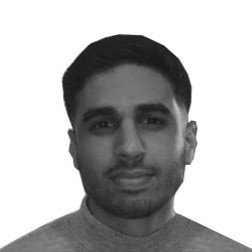
Hassan graduated with a Master’s degree in Chemical Engineering from the University of Chester (UK). He currently works as a design engineering consultant for one of the largest engineering firms in the world along with being an associate member of the Institute of Chemical Engineers (IChemE).