Multiple principal element alloys (MPEAs); metals composed of three or more elements, combine strength, corrosion resistance and thermal stability in a single material. A team led by Sanket Deshmukh at Virginia Tech has applied an explainable AI framework to design a new FeNiCrCoCu MPEA with improved mechanical properties, replacing much of the trial-and-error approach that has been the norm in industry. The research was published npj Computational Materials and can be found here:
Wang, F., Iwanicki, A. G., Sose, A. T., Pressley, L. A., McQueen, T. M., & Deshmukh, S. A. (2025). Experimentally validated inverse design of FeNiCrCoCu MPEAs and unlocking key insights with explainable AI. Npj Computational Materials, 11(1), 124. https://doi.org/10.1038/s41524-025-01600-x
Standard AI models predict outcomes without revealing their reasoning. By contrast, the explainable AI framework used here incorporates SHapley Additive exPlanations (SHAP) to identify how element concentrations and local atomic arrangements affect properties such as unstable stacking fault energy and bulk modulus.
The team’s workflow combines two computational models: a stacked ensemble machine learning (SEML) model for predicting unstable stacking fault energy (USFE), and a one-dimensional convolutional neural network (CNN) for bulk modulus prediction. Evolutionary algorithms then explore millions of compositions. Top candidates were synthesised as FeNiCrCoCu alloys, and X-ray diffraction confirmed single-phase face-centered cubic (FCC) structures. Hardness and Young’s modulus tests matched the model’s predictions. Sanket Deshmukh stated:
“This work demonstrates how data-driven frameworks and explainable AI can unlock new possibilities in materials design, by integrating machine learning, evolutionary algorithms, and experimental validation, we are not only accelerating the discovery of advanced metallic alloys, but also creating tools that can be extended to complex material systems such as glycomaterials—polymeric materials containing carbohydrates.”
Predicted bulk moduli reached up to about 190 GPa, compared to roughly 155 GPa for baseline alloys, indicating a gain in stiffness and resistance to deformation. Moving from empirical testing to model-guided design cuts development time and reduces experimental cost. Fangxi “Toby” Wang, postdoctoral associate in chemical engineering and researcher on the project said:
“Leveraging explainable AI accelerates our understanding of MPEAs’ mechanical behaviors. It could transform the traditional expensive trial-and-error materials design into a more predictive and insightful process, our design workflow, combining advanced machine learning and evolutionary algorithms, provides interpretable insights into materials’ structure-property relationships, offering a robust approach for the discovery of diverse advanced materials.”
The project involved partners at Johns Hopkins University and Virginia Tech’s GlycoMIP platform, including Tyrel McQueen, Maren Roman and Allana Iwanicki, who led the synthesis and mechanical testing.
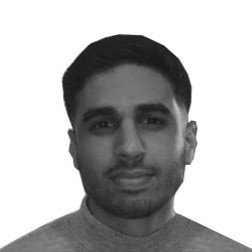
Hassan graduated with a Master’s degree in Chemical Engineering from the University of Chester (UK). He currently works as a design engineering consultant for one of the largest engineering firms in the world along with being an associate member of the Institute of Chemical Engineers (IChemE).