When chemists plan a new synthesis, knowing the transition state; the highest-energy configuration of atoms at the peak of a reaction’s energy barrier, where old bonds are partially broken and new bonds are partially formed, guides them toward the right catalysts and conditions. Locating that transition stated and therefore, the correct configuration of a chemical reaction has meant hours or days of quantum-chemistry computations using huge amounts of energy. React-OT, a machine-learning approach developed by researchers at MIT, reduces that time to roughly 0.4 seconds while improving accuracy by about 25 %. The research, which was published in Nature Machine Learning, can be found here:
Duan, C., Liu, G.-H., Du, Y., Chen, T., Zhao, Q., Jia, H., Gomes, C. P., Theodorou, E. A., & Kulik, H. J. (2025). Optimal transport for generating transition states in chemical reactions. Nature Machine Intelligence, 7(4), 615–626. https://doi.org/10.1038/s42256-025-01010-0
What is React-OT?
React-OT (Reaction Optimal Transport) is trained on a dataset of 9,000 quantum-calculated reactions, used in both organic and inorganic cases. Instead of relying on random starting points, it begins with a linear interpolation of atom positions; from reactants to products, placing it much closer to the true transition state right away.
Most existing models generate dozens of candidate structures from random guesses, then filter the results through a separate confidence network. React-OT uses optimal transport theory to shift atomic positions along the most efficient path between reactants and products. This sharper initial guess cuts the required inference steps to about five and removes the need for an extra validation stage. Heather Kulik, the senior author of the study, and the Lammot du Pont Professor of Chemical Engineering, a professor of chemistry said:
“We’d like to be able to ultimately design processes to take abundant natural resources and turn them into molecules that we need, such as materials and therapeutic drugs. Computational chemistry is really important for figuring out how to design more sustainable processes to get us from reactants to products,”
In benchmark tests, React-OT achieved a median root mean square deviation of 0.053 Å and a median barrier-height error of 1.06 kcal/mol—all in under half a second per reaction.
The team also evaluated React-OT on polymer-related reactions where only one segment of a macromolecule is active. The model’s accuracy was maintained, indicating it can generalise to larger systems without retraining . According to Markus Reiher of ETH Zurich, faster transition-state searches not only speed up catalyst design but also cut the energy footprint of high-throughput studies. Markus Reiher was not an author or involved in the study.
“To quickly predict transition state structures is key to all chemical understanding, the new approach presented in the paper could very much accelerate our search and optimization processes, bringing us faster to our final result. As a consequence, also less energy will be consumed in these high-performance computing campaigns. Any progress that accelerates this optimization benefits all sorts of computational chemical research.
By trimming computation time and resource use, React-OT could make high-throughput reaction screening more sustainable. Chemists can now estimate activation barriers and compare pathways in a few seconds, rather than waiting days.
The MIT team is extending React-OT’s scope to include elements such as sulfur, phosphorus, and silicon, and plans to embed the model in existing automation platforms for reaction discovery.
To promote adoption, the researchers have launched a web application. Users upload reactant and product structures and receive a predicted transition state along with an estimated energy barrier.
The study was led by Heather Kulik, the Lammot du Pont Professor of Chemical Engineering at MIT, with former MIT graduate student Chenru Duan (now at Deep Principle), former Georgia Tech graduate student Guan-Horng Liu (now at Meta), and Cornell University graduate student Yuanqi Du as co–lead authors. The work was supported by the U.S. Army Research Office, the Air Force Office of Scientific Research, the Department of Defense Basic Research Office, the National Science Foundation, and the Office of Naval Research.
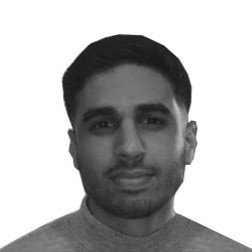
Hassan graduated with a Master’s degree in Chemical Engineering from the University of Chester (UK). He currently works as a design engineering consultant for one of the largest engineering firms in the world along with being an associate member of the Institute of Chemical Engineers (IChemE).